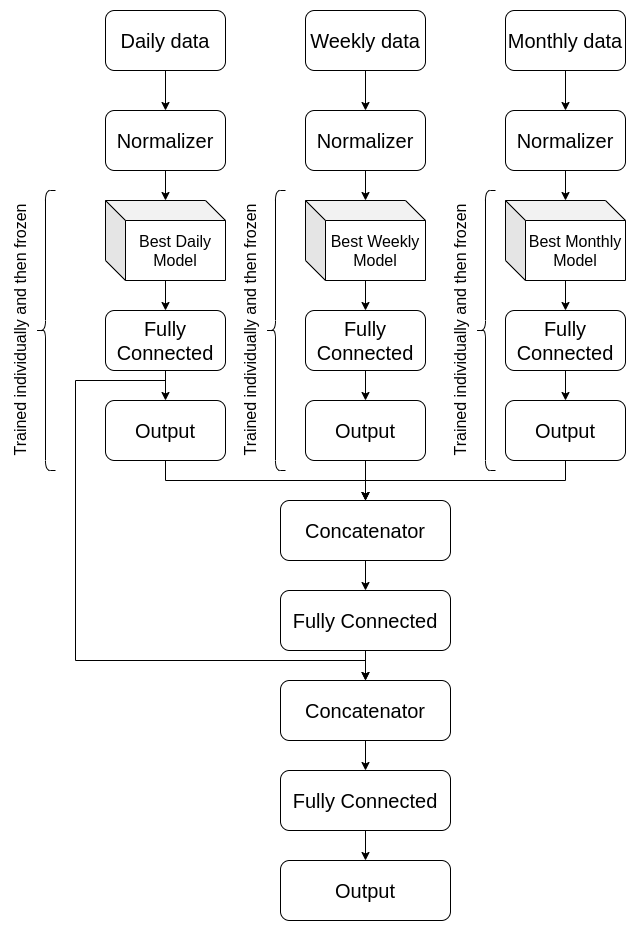
HSC-RNN-AM
Crude oil, as a prerequisite for many industries, is vital in today’s world. In this regard, predicting its future price is crucial for many purposes. Even though plenty of research has been done in this field with many methods, such as evolutionary algorithms, neural networks (NN), and other machine learning techniques, because of the extremely unpredictable nature of crude oil prices, the outcomes are not satisfactory. This study employs 39 features for oil price prediction and proposes a hybrid architecture for deep NNs (DNN) to take advantage of features in different periods. Attention-based DNNs are utilized in the proposed architecture, and the comparisons are based on the mean absolute error. The results show that (1) attention-based DNNs are useful for forecasting the crude oil price with many features, and (2) the proposed architecture can enhance the accuracy of previous models.